In EFFORTE, August 2017 was time to prepare and finish 4 deliverables. These deliverables were strongly focused on Big Data and applications using it. In the project one key objective is to describe all meaningful and potential forestry related Big Data and to create decision-support models, such as forestry applications based on these data. They aim to increase cost-efficiency and new business opportunities in forest operations – sustainably, of course.
Many methods, one database
Deliverable D3.2 Project database surveyed and compiled all the Big Data and methods which have been utilized and developed in EFFORTE so far. All in all, 32 different Big Data sources and 15 methods were listed. As the method development continues in EFFORTE, new data sources and methods will be added to the project database, which will offer the base information and possible links to data sources and methods with updates.
Big Data plus maps equals new tools
Some of the Big Data sources and methods were described in more detail in the rest of the deliverables, which focused on trafficability and precision forestry themes. As the trafficability issue is very important in practical forest operation planning and operations, map-based decision-support systems have been developed. Report opens the structures of static trafficability map, depth-to-water (DTW) map and dynamic trafficability map, which all are utilizing multisource data, such as LIDAR data from forests and terrain together with intelligent data analysis.
The DTW map (in D3.3 & 3.4) illustrates how far moving soil water is from the soil surface. It provides decision support for the forest planner or operator to ease the planning and forest operation with higher productivity and less soil disturbance. This suites well for many applications as the map is intuitive and easy to explain. Read more about the map from here.
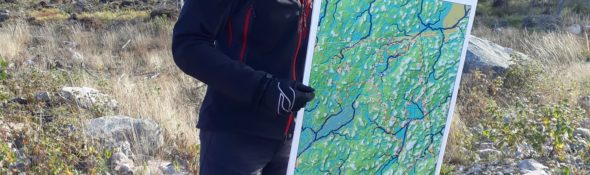
Static or dynamic? Trafficability maps for all needs
The static trafficability maps (in D3.3 & 3.4) are user-friendly and all forest areas may be mapped with a thematic class. The map provides good support for deciding the season for site operations. On the other hand, future dynamic trafficability maps may provide more accurate information in defining exact timing for the operation or in finding the most appropriate routes for logging trails. Presented trafficability models are however rather specific and may require country specific modifications.
Precision forestry needs support tools too
The last of four deliverables (D3.6) presented how to utilize Big Data sources in precision soil preparation and other regeneration activities. Ultimate target for precision forestry (PF) is to increase forest growth and improve efficiency of soil preparation and regeneration methods. In addition to terrain data obtained by LIDAR, also spatial harvester data is part of the base data sources in this. PF support tools can detect the no-go areas, aid in selecting more precise scarification method and tree species for the regeneration as well as monitor the proportion of rotten wood at the site, for example.
Methods, tools and data for true needs
According to the industry all presented decision support tools have been an important step towards more precise decision support with more accurate information on terrain and forest. An interlinkage to the weather as a dynamic component has increased its importance in development of decision-support systems for the practice. Importance of spatial soil data is stated to be in high concern and essential addition to DS systems.
Prepared deliverable reports gave a general understanding of how Big Data have been or can be used in forestry operations now and in close future. Reports present also the benefits of utilizing the data in practice. Countries have differences in data accuracy, availability and practical forestry demands, thus there are differences in data and methods between countries.